As businesses worldwide take the reins to harness the power of data and AI initiatives, they are finding intelligent ways to store and manage their growing data banks. Gartner forecasted by 2025, 90% of new data and analytics deployments will be carried out via an established data ecosystem, causing consolidation across the data and analytics market. In this new world of automation and Artificial Intelligence (AI), distinguishing between data lakes and data warehouses can be pivotal to achieving a solution that benefits your business. While both serve as foundational technologies for data management, their roles differ significantly, especially in the context of AI and Machine Learning (ML).
Both data storage solutions offer unique advantages that can directly impact the efficiency and accuracy of AI and ML operations, making this decision an essential factor in leveraging data for business growth. This article breaks apart both systems to highlight the differences between data lakes and data warehouses, how they integrate with AI and ML, and the benefits they offer to businesses looking to gain an edge in today’s data-driven landscape.

Source: Gartner
Key Differences: Data Lakes vs Data Warehouses
Data lakes and data warehouses serve distinct purposes within an organisation’s data strategy. While both serve as foundational technologies for storing and managing data, they cater to different needs. A data lake is a vast repository that stores data in a raw, unprocessed form. It offers flexibility and scalability, which is ideal for storing various data types, including structured, semi-structured, and unstructured data. This flexibility is crucial for AI and ML applications that often require access to large volumes of diverse data types for training and data analysis.
On the other hand, a data warehouse is a structured environment optimised for querying and reporting on cleaned and processed data. Unlike data lakes, data warehouses impose a predefined schema on the data, making it easier to perform business intelligence tasks and generate insights from structured data. The structured nature of data warehouses is beneficial for tasks that require high performance and reliability, such as operational reporting.
The Role of Data Lakes in AI and ML
Data lakes can be invaluable for AI and ML because they store vast amounts of raw data. AI models often require access to unprocessed data to learn from patterns and anomalies, and data lakes provide the necessary environment for such processes. By enabling the storage of varied data types, including images, videos, and log files, data lakes empower ML algorithms to develop more accurate and robust models.
Key Advantages:
- Scalability and Flexibility: Data lakes can store extensive raw, unstructured, and semi-structured data, essential for comprehensive AI and ML model training and experimentation.
- Cost-Effectiveness: By storing data in its raw form, data lakes reduce the need for extensive preprocessing, lowering costs and enabling reuse across multiple AI and ML projects.
- Support for Advanced Analytics: Data lakes integrate seamlessly with AI and ML tools, enabling advanced analytics, real-time processing, and accelerated model training for more dynamic and accurate AI applications.

The Role of Data Warehouses in AI and ML
When working with structured data, data warehouses are essential for AI and ML. They offer a streamlined approach to building predictive models and deriving valuable business insights. Their ability to support advanced analytics, real-time processing, and consistent, high-quality data makes them invaluable in AI.
Key Advantages:
- Consistent Data: Data warehouses ensure that AI and ML models are trained on clean, structured data, reducing errors and leading to more accurate outcomes.
- Data Access and Query Performance: Built for fast data retrieval, data warehouses enable quick access to large datasets, speeding up the AI model development process.
- Support for Real-Time Processing: Data warehouses integrate with advanced analytics tools to allow real-time processing. This enables AI models to respond quickly to live data inputs.
Which is Better for My Business: a Data Lake or a Data Warehouse?
When deciding between a data lake and a data warehouse, businesses must consider several factors, including the nature of their data, the specific requirements of their AI and ML projects, and their long-term data strategy. Data lakes are generally more suitable for organisations with large volumes of unstructured or semi-structured data, where flexibility and scalability are crucial.
On the other hand, if an organisation’s primary focus is on structured data and requires fast, reliable access to refined datasets, a data warehouse may be the better option. Additionally, businesses must consider their existing infrastructure, the skill sets of their data teams, and the anticipated growth of their data volumes. Balancing these factors will help determine which storage solution aligns best with the organisation’s AI and ML objectives.
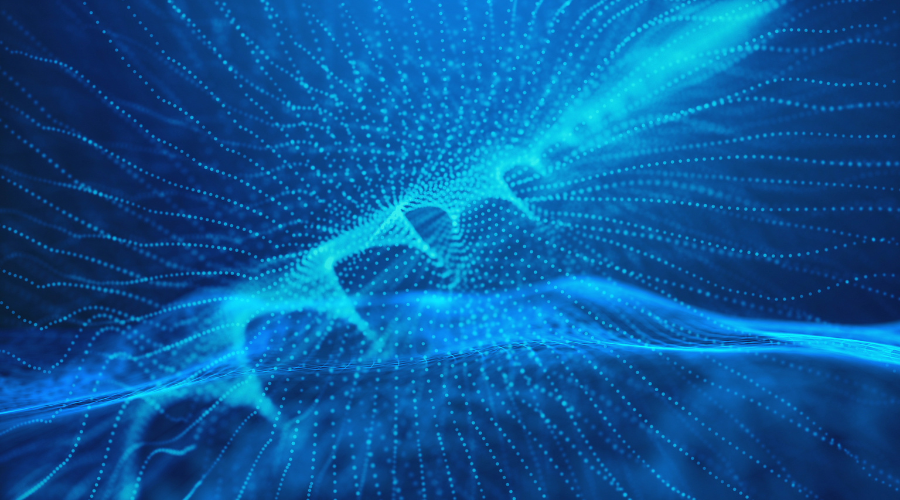
Conclusion
Choosing between a data lake and a data warehouse is not a one-size-fits-all decision. Both have their distinct advantages, particularly when it comes to supporting AI and ML functions. By understanding the differences and aligning the choice with their specific needs, businesses can make informed decisions that drive their AI and ML efforts forward. In the competitive landscape of the Australian industry, the right data strategy could be the key to unlocking significant growth and innovation.
MakeSense Can Help You Choose Between a Data Lake or a Data Warehouse
MakeSense can get your data strategy up to spec for your AI and ML operations. If you’re ready to enhance the quality of your data for AI outputs, MakeSense specialises in implementing data lakes or data warehousing for your AI and data solutions, including automation, deep learning, and data engineering. Our experts deliver tailored solutions to support strategic decisions and drive your company’s future growth.
Visit our AI and Data Services page to learn more about how we leverage AI and data analytics to achieve your strategic goals.
Related Blogs
AI’s value relies on the quality of your data
The challenges and opportunities of using advanced AI for data analysis
Building a data-driven culture: The importance of data governance